Second part of: Mining PubMed for Drug Induced Acute Kidney Injury
When I wrote “Mining PubMed for Drug Induced Acute Kidney Injury”, my intention was to start exploring the use of PubMed for knowledge discovery in the fields of drug safety and pharmacovigilance. But to discover new knowledge, you need to know what is already known, what has been discovered already.
Using our example of drug induced acute kidney injury (AKI), if we want to discover new associations, we should be aware of which drugs are known to increase the risk of renal damage, or to worsen renal function on an already impaired kidney.
For marketed, prescription drugs, we can use the FDA labels as a reference of what adverse reactions are already known for a specific product, and check them against our PubMed search, for knowledge discovery.
How can we reach that goal? First, it is helpful to know that the FDA provides us with the labels of all approved products, in xml format. To download FDA labels click here.
To understand this approach, we need to talk about a variety of concepts and how they can help us to reach our objectives:
Semantic Web
The Semantic Web is a Web 3.0 technology. It is a way of connecting data between entities or systems that allows for rich, self-describing interactions of data available worldwide across the Internet. Nowadays, the majority of information provided by the Internet is delivered in the form of web pages. These documents are linked each other through the use of hyperlinks. Humans or machines can read these documents. But machines, other than finding keywords on a page, have difficulties extracting any meaning from these documents.
The semantic web will open the web of data to artificial intelligence processes, it seeks to encourage people to publish their data in an open standard format, at the same time that encourages Internet users to analyze these data and gain knowledge.
The Graph Database
The graph database is the way the semantic web stores data. The Resource Description Framework, or RDF, constitutes the building blocks for forming the web of semantic data, and it defines a type of database which is called a graph database.
Data can be stored in the form of triples. A triple describes the breaking of an RDF statement into its 3 constituent parts: the subject, the predicate (or property), and the object of the statement. For example, we want to define the color of a capsule for a medicinal product:

In terms of this simple graph, the subject is the capsule; the predicate (or property) is color; and the object is red. That’s why this is called a “triple”, and the information is stored in triples.
Semantic Modeling
RDF offers a flexible, graph-based model for recording data that is interchangeable globally, and this is the beauty of it. However, it does not offer any means of recording semantics, or meaning.
We want to include semantics in data, for the purpose of knowledge integration. One of the most important benefits of adding semantic meaning to our data is that it can be bridged across domains of knowledge automatically. For example, suppose we have two websites, one of them stores information about product labels, including all adverse reactions, and the other stores information about treatments given to a specific group of patients. Although these 2 sites have been created independently, the information they provide is complementary.
In principle, any sharing of data between the 2 sites cannot be done, in principle, by joining tables in their databases. This is because they have been designed independently, and because they are using different database server systems, which are not cross-compatible. This type of information interchange across incompatible, independently defined data systems takes time, money, and human contextual interpretation of the different sources of data. It is also limited to these 2 websites / datasets. Any further additions to their knowledge from elsewhere would require a similar effort.
With the introduction of semantics and RDF, all this is much easier to do. How do we model the two site scenario using semantic modeling? To begin with, the 2 sites need to apply a common, standard vocabulary (a collection of terms with a well-defined meaning that is consistent across contexts). This can be done if the two sites adopt the same ontology (to define contextual relationships behind a defined vocabulary), for expressing the meaning behind the data they expose, and publishing the data on an endpoint which can be queried, so that the sites can communicate with each other across the web.
NOTE: Currently you can download from the Web thousands of databases encoded as triples. Among the largest ones we highlight DBpedia, which is the triple-store version of Wikipedia.
Example Applied to Drug Safety – Drug Induced Acute Kidney Injury
In this example we can see how different databases containing partial health-related information that are conceptually interconnected, can be linked for knowledge discovery.
Data from SNOMED (global common language for health terms), MeSH (Medical Subject Headings, a comprehensive controlled vocabulary for the purpose of indexing journal articles and books in the life sciences), SIDER (Side Effect Resource), DailyMed (drug brand names and FDA product labels), ClinicalTrials.gov (web data source for clinical trials), DrugBank (comprehensive data about medicinal products), and the Diseasome (integrated database of genes, genetic variation, and diseases), along with any patient record data, or even PubMed data can be interlinked and queried. It opens a myriad of opportunities. And this is just a small example of what we can do.
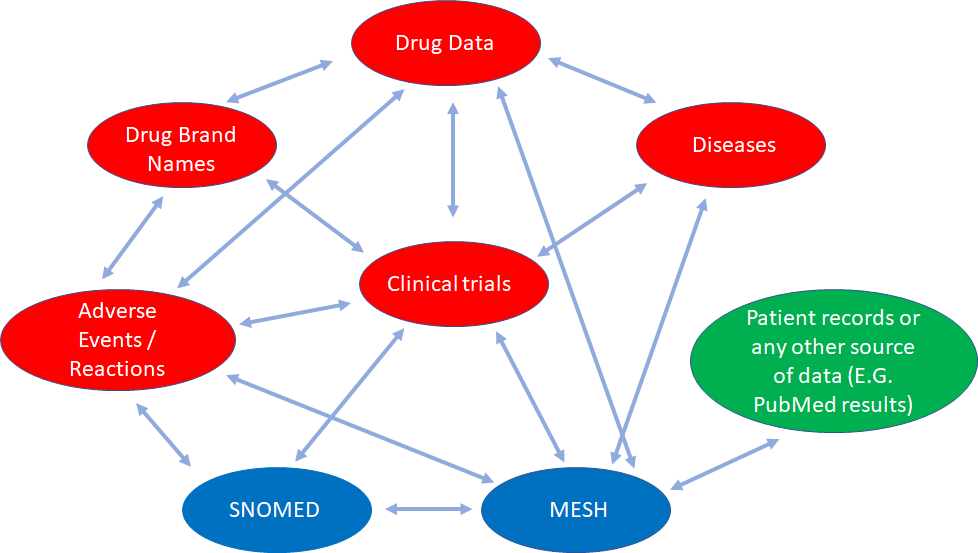
Graphic-Based, Triple-Store Browser
We are going to use a tool to display visual graphs of subsets of a store’s nodes and their links. It is an interactive tool for browsing, querying, and editing triple-stores, also known as graph databases.
On the previous post of this series, we found 8,916 PubMed abstracts for the search of drug induced acute kidney injury. We downloaded all the abstracts as an xml file. Some applications are able to obtain triples from them, in such a way that allows us to analyze them graphically. In this case, we got 2,705,300 triples from the mentioned PubMed results.
A simple example of it is shown on the next picture. We wanted to know how many abstracts were talking about “acute kidney injury”. By searching that keyword, the tool delivered 599 nodes (abstracts) and 300 links:
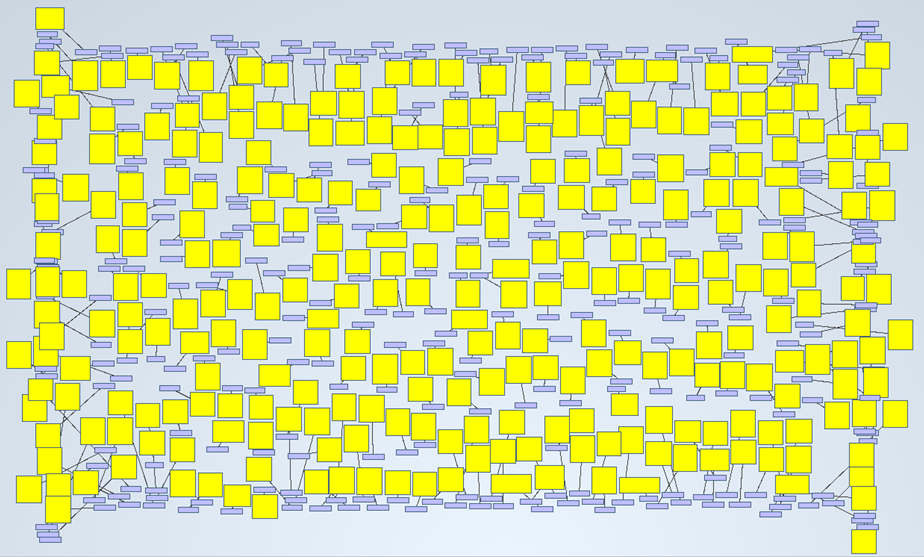
If we zoom in, we will see this:
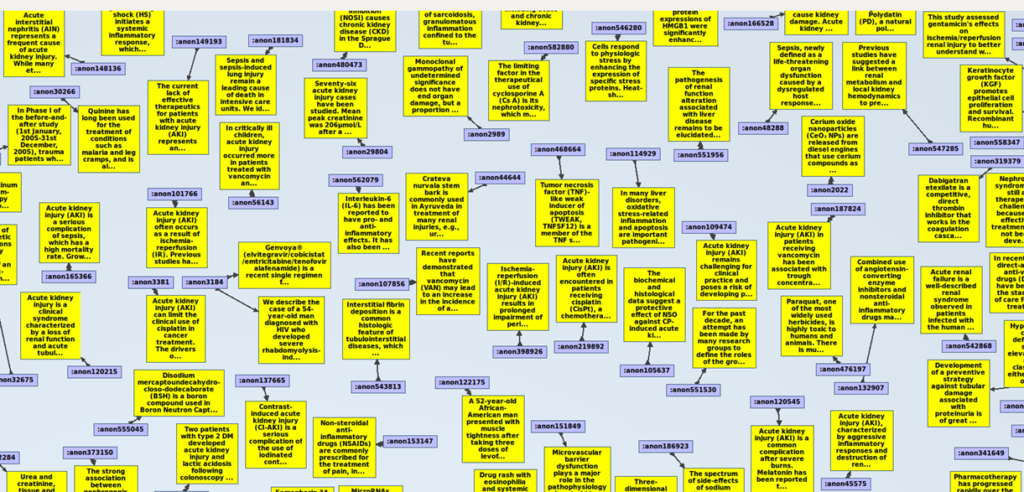
Let´s see how the triples look like in this graph database. Remember that we have converted the xml file into a triple-store, and that triples consist of Subject, Predicate, Object. Following there is a list of the 83 predicates extracted from the PubMed xml file we are using for this example, in alphabetical order:

Follow this link if you want to learn more on PubMed XML Element Descriptions and their attributes.
These predicates are the properties of each one of the articles we retrieved. The subject would be a unique identifier for each article, the predicate is one among the previous list, and the object is the value of the predicate for that specific article.
Some sample triples from the dataset are shown here:
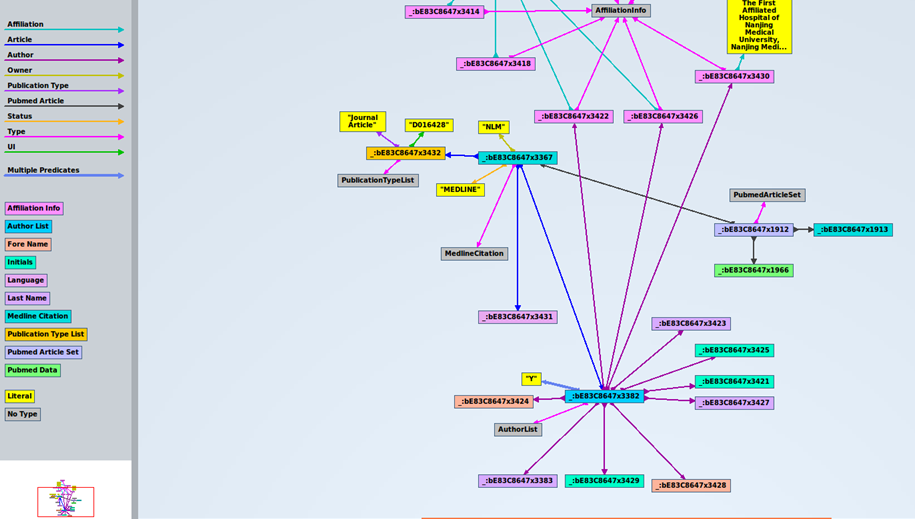
Next, we can see the first triples of the dataset, where column “s” is for subjects, “p” is for predicates, and “o” is for objects:
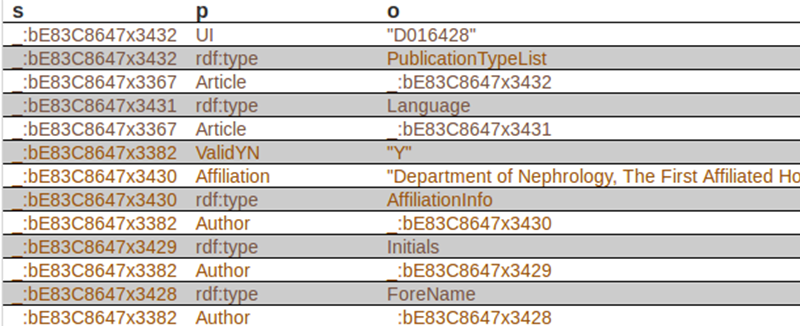
The first subject is _:bE83C8647x3432, corresponds to a specific article. The corresponding predicate (property) is UI, and the object is D016428. In case you want to know, this element is used to identify they type of article indexed by MEDLINE. There is a code for each type of article. “D016428” is the code for the object “Journal Article”. Records may contain more than one publication type. In our case, this record contains just one publication type. In xml, it looks like this:
<PublicationTypeList>
<PublicationType UI=”D016428″>Journal Article</PublicationType>
….
</PublicationTypeList>
When we click on ” _:bE83C8647x3432″, this is what we get all the statements with that code as the subject. It shows the predicates associated to it, and the objects associated to the predicates:
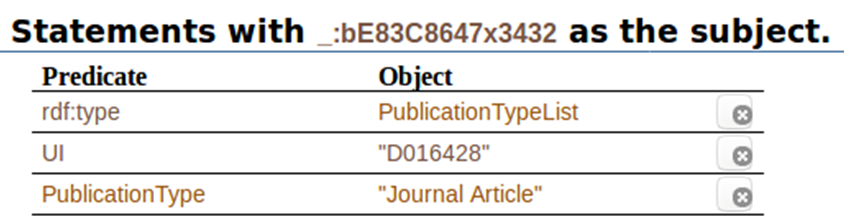
In this post, we have talked about knowledge graphs, semantic web, triples, and have shown some of them, applied directly to our PubMed search on drug induced acute kidney injury. The next post will show more about it, and more results from including other, completely different, sources of data.